From facial recognition software on our smartphones and email spam filters to product recommendations on e-commerce sites, machine learning is improving various areas of our lives.
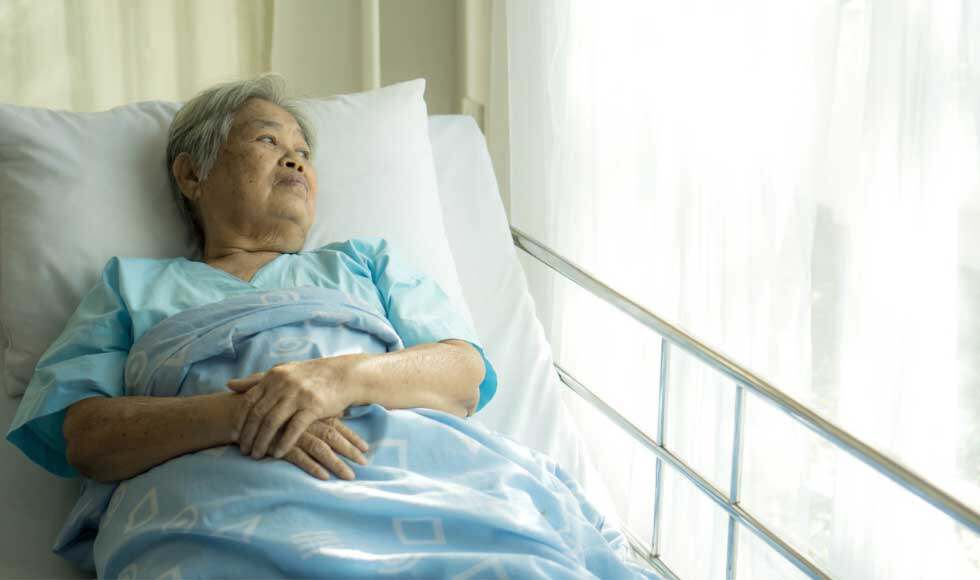
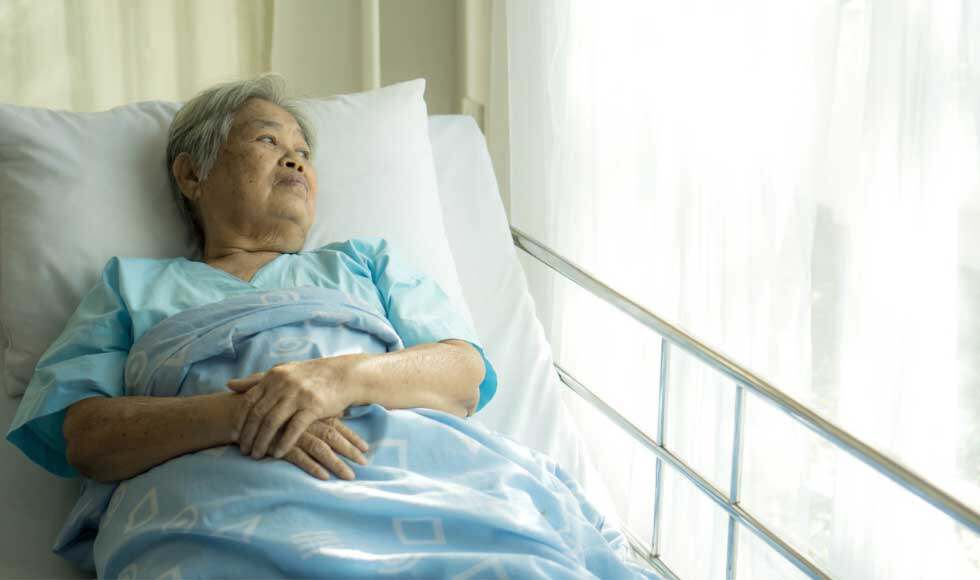
DeGroote School of Business researcher Manaf Zargoush is using his data analytics expertise, machine learning, and optimization to advance the management and prevention of a healthcare issue called Alternate Level of Care (ALC).
Each year in Canada, ALC applies to about one-fifth of the 3 million hospital patients seeking acute care who get stuck in a hospital bed because the homecare support or long-term care facility they need is unavailable. Their care journey is interrupted. They no longer require the level of care a hospital provides but still need additional care and support. ALC patients are primarily older adults. As they wait to be discharged, the hospital bed they occupy cannot be used by another patient.
“ALC has a domino effect,” says Zargoush, associate professor of Health Policy and Management.
“Research shows that during the waiting time for their next care setting, an ALC patient’s health deteriorates. This can lead to readmission, which is another critical issue in healthcare, and happens because, most likely, we did not make a good decision about the patient’s care.
“Many researchers believe that one of the main reasons, if not the primary reason, for the long healthcare waiting times in Canada is the ALC phenomenon. We have people in the emergency room waiting to get in the hospital. But because the bed is occupied as an ALC, they have to wait. The emergency room keeps getting crowded, and many surgeries are cancelled. So, everyone, with no exception, will be impacted by improved decision-making regarding ALC.”
USING MACHINE LEARNING TO MANAGE ALC
Zargoush says that part of the reason the healthcare system encounters challenges such as ALC is that it lags in using data to make predictions for improving decisions. That’s where his research comes in. Using big data to predict things such as a patient’s future health status or the cost of potential care decisions, Zargoush says, can lead to better decision-making, reduced costs, reduced waiting times, and improved health care.
A rich data set that includes 18 years of information about almost all ALC patients in Ontario informs Zargoush’s ALC research. On one ALC project, Zargoush worked with a postdoctoral scholar, a PhD student, and experts from Trillium Health, the University of Toronto, and the University of Alberta. This interdisciplinary team investigated how to improve discharge planning for older ALC patients. They designed a model that predicts the health status of patients and the associated healthcare system cost of transitions.
“Imagine I have two patients, and I have one long-term care bed becoming available,” he explains. “Which patient should I assign to this long-term care bed? The solution, which is common practice right now, is first come, first serve. But what if I tell you the patient who came later, based on the prediction that I’m making about the future, needs that bed more severely than the other patient? Does that information not change your decision? It does, right?
“So, we look at the patient’s characteristics, what happened to them, and what their health status was at different points in time, and this is how we use artificial intelligence to learn from what has happened before in terms of our decision-making right now and its impact in the future. We’ve shown that this kind of decision-making outperforms many other decision-making paradigms, including first come, first serve.”
In another ALC project, Zargoush is investigating the prevention of ALC in acute hospitals by providing at-risk individuals with earlier access to community resources. To do this, Zargoush develops machine learning predictive analytics using big data. He creates profiles of older adults at risk of ALC by looking at ALC risk factors patients experienced during the previous two years. These risk factors may include a history of hospitalization and functional and cognitive impairment.
THE APPLICATION OF ALC RESEARCH
In a homecare or long-term care community setting, these patient profiles can then inform the creation of individualized service packages with patients, their family members, and care providers that will help prevent hospital visits and avoid harm and decline if a patient goes into the hospital. Meanwhile, in a hospital healthcare setting, a computer can use Zargoush’s algorithm in tandem with a patient’s record to inform various care and transition decisions. Policymakers can also use the research to prioritize budget allocation and long-term decision-making.
“The end goal of medicine and healthcare is to do targeted intervention,” says Zargoush. “We want to know what we should do for what people. Right care, right time, right person. To me, data is history, and we can learn from history to make our future better. Using data and machine learning to solve problems is going to revolutionize the healthcare industry and medicine entirely.”